Top Hamster Breeds for Compact Living Spaces When it comes to choosing a pet for compact living spaces, hamsters are
Continue reading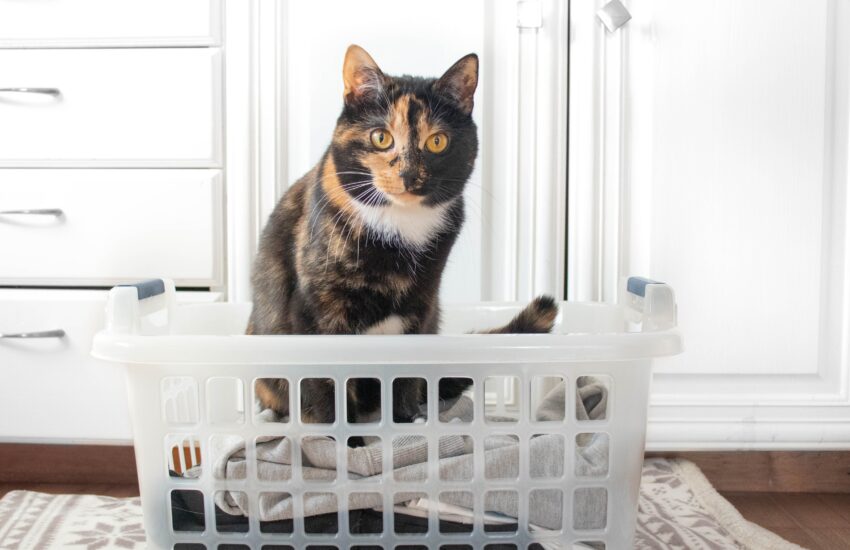
Top Hamster Breeds for Compact Living Spaces When it comes to choosing a pet for compact living spaces, hamsters are
Continue readingBest Hamster for Learning Tricks Understanding Hamster Breeds for Tricks When it comes to choosing the **best hamster for learning
Continue readingTop Hamsters for Children’s Learning Hamsters are not just adorable pets; they can also be remarkable learning tools for children!
Continue readingBest Pet Hamsters for All Ages Hamsters make excellent pets for individuals and families of all ages due to their
Continue readingBest Hamster Breeds for Easy Handling Hamsters are popular pets known for their adorable appearance and playful nature. They are
Continue readingBest Hamster Breeds for Introductions When considering adding a furry companion to your home, **hamsters** are excellent pets. Known for
Continue readingBest Hamster for Learning Responsibility Owning a pet is a wonderful way to learn responsibility, and hamsters are particularly suitable
Continue readingBest Hamster Breeds for Indoor Activities When it comes to choosing the perfect pet for indoor activities, hamsters often top
Continue readingBest Behaved Hamster Types When it comes to choosing a pet, hamsters are often a favorite for families, children, and
Continue readingBest Hamster Breeds for Families with Allergies If your family is considering getting a pet hamster but has allergies, it’s
Continue reading